UQ in Earth Science Data#
This article is part of a series: Subjectivity in Earth Scinece Data.
Subjectivity in Earth Science Data#
Subjectivity is a distinct type of uncertainty related to Earth science data. It is inherently linked to the role human agents play in information processing chains resulting in typical Earth science products, such as geological maps and models of processes taking place in the Earth. Compared to other types of uncertainty present in information processing chains, it is particularly challenging to quantify the amount of subjectivity present in information processing.
Subjectivity and its Relation to Uncertainty#
In the following discussion, we consider data as quantitative units of coded information. We consider information in the sense of knowledge which is cognitively understandable to human agents. Information or knowledge exists in the brain of a human agent. To share it or store it outside the human brain, it has to be coded into data. For example, this can be done by coding information into letters, words, and sentences, into sound sequences with temporarily varying frequency and amplitude, or into binary sequences. Information coding to produce data is done following specific rules which are ideally precisely and accurately determined. In practice, accurate and precise information coding can be challenging, e.g., when sharing information on how to do big wave surfing with someone who never tried surfing before . Unlike information, data can be transmitted and received by other human agents. To retrieve information from the received data, these data have to be decoded following the same rules as used for information coding (Fig. 73).

Fig. 73 Simplified information processing chain between human agents.#
In modern Earth sciences, data can also be produced by technical devices sampling the environment discretely. At the beginning of the information processing chain, information is replaced by the environmental reality which is coded into data following specific algorithmic rules defining the technical device and its coding precision and accuracy. Since both are practically limited, the produced data always carry uncertainty in the form of a probabilistic deviation from their optimal or correct quantitative states [JCGM, 2008]. The outcome is discrete data usually stored and transmitted in binarized form. Despite the utilization of technical sensors on the data production side, humans are involved in the information retrieval from such data when turning data into information (Fig. 74).
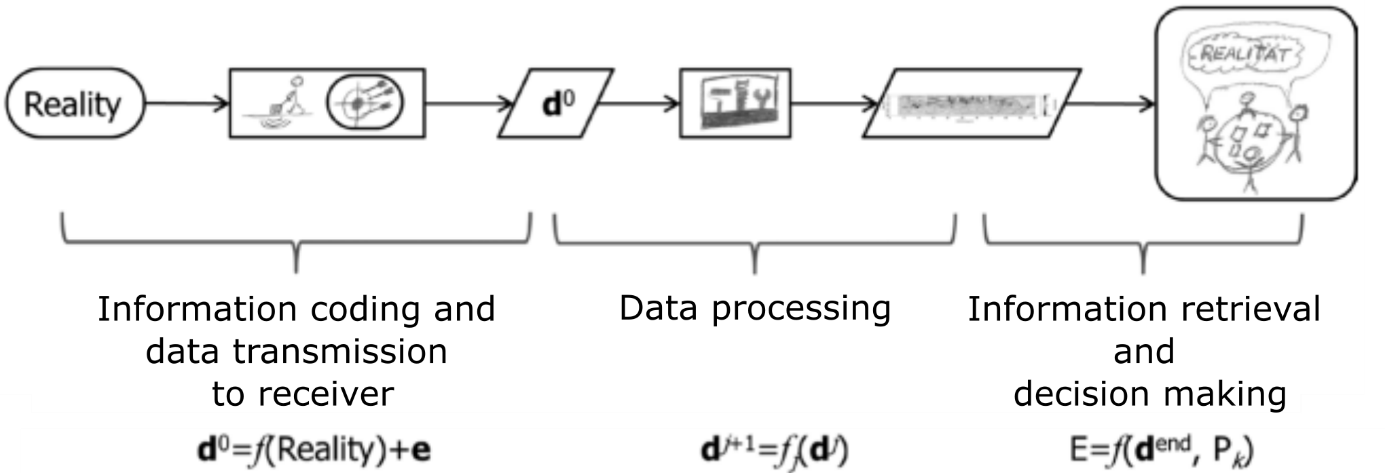
Fig. 74 Workflow from information coding to information retrieval and decision making when using a technical device for data production ([Paasche et al., 2020], modified).#
Information processing chains comprising information or reality coding, data transmission, data reception, and information retrieval are prone to uncertainty. This becomes obvious if a mismatch between coded and retrieved information exists. Reasons for that can be manifold. For example, coding rules may have been applied improperly. This may result in poor coding results, e.g., with regard to accent, wording, and incorrect grammar utilization when coding information into a sound sequence using the rules of a language. Consequently, aspects of the information may be lost or biased during the coding. This can cause irreversible information distortions even when the data receiver has good decoding skills, e.g., is a native speaker of the language used. Data may interfere with environmental noise, e.g., spoken words and sentences may be superimposed by loud environmental noise when transmitted.
Classic Types of Uncertainty – Aleatory, Epistemic, Ontological#
The degree up to which information coding has been done precisely and accurately defines the most optimistic limits for information retrieval. This applies to every datum in a data set. A datum without its aleatory uncertainty (e.g., [Der Kiureghian and Ditlevsen, 2009]), i.e., a quantitative statement about its coding accuracy and precision, is incomplete ([JCGM, 2008]). If information retrieval exceeds the aleatory uncertainty of a datum, it is over-interpreted. To enable correct information retrieval, it is therefore an essential task of the information coder to quantify the aleatory uncertainty of the produced data and share this information together with the data. If data are produced by technical devices following algorithmic procedures, this is largely possible by suitable experimental design, albeit often expensive [Paasche et al., 2020]. If information coding is done inside a human agent’s brain, the ability to provide realistic aleatory uncertainties for the coded data is not given, since the human agent cannot quantify biases and random effects in the coding routines applied. The most important reason for this is that a human agent holds only a limited representation of the world based on their own experience, including information and information coding routines [Hanea et al., 2022].
Practical limits for information retrieval are usually even lower than the aleatory data uncertainty at the coding step. Distortions during data transmission, reception, and information retrieval procedures might add uncertainty to the information retrieval. Without knowledge about aleatory data uncertainty at the data reception, human agents fill this knowledge gap by a guess based on their experience, i.e., a more or less consciously reflected data uncertainty model. Inexperienced human agents face a high risk to follow a poor guess making their information retrieval highly uncertain. Particularly for laymen not experienced with the kind of data and the information retrieval from these data, knowledge of aleatory data uncertainty is essentially required to guide their information retrieval procedures in order to keep the uncertainty added in the information retrieval step small.
It should be noted that the transformation of data, e.g., by means of a simple frequency filter or a deep AI system, into a subsequent data instance prior to information retrieval can emphasize or suppress specific aspects of the data which are a priori considered to be important or disturbing for correct information retrieval, respectively (Fig. 74). Such transformations convert data into representations which are more easily cognitively accessible for human agents. This apparently makes the final human information retrieval step simple even for users inexperienced with the data. Nevertheless, any data conversion can be understood as an information retrieval from data in combination with a further coding step. This is analogue to an, in most cases, non-linear distortion of the original coding rules. Such data processing does not result in a reduction of uncertainty [Paasche et al., 2022]. It rather reduces quantifiable aleatory uncertainty related to information coding accuracy and precision on costs of increasing uncertainty related to the choice and suitability of “the right” transformation method. This methodology related uncertainty is known as ontological uncertainty [Lane and Maxfield, 2005].
When we consider data as coded units of information, resulting data sets are discrete. Discrete data comprise gaps of information between adjacent data. This adjacency can be related to space or time resulting in band-limited data sets with limited spatial and temporal resolution. When imaging reality into data, further band-limitations can result from limitations of the sensor construction, e.g., the sensitivity of the sensor to a narrow frequency spectrum. This results in knowledge gaps about information beyond the resolution of the data set or physical sensor limitations. The existence of such knowledge gaps results in epistemic uncertainty [Der Kiureghian and Ditlevsen, 2009]. Its existence in a data set is usually known when retrieving information from data sets but cannot be overcome without the consideration of further data, e.g., in the sense of data integration or information fusion, or by applying a model considered trustworthy [Paasche et al., 2020]. Information fusion strives to convert epistemic uncertainty into aleatory uncertainty of the additionally considered data, whereas application of a model converts epistemic uncertainty into ontological uncertainty.
summarizes the classical data uncertainty models comprising aleatory, epistemic and ontological uncertainty and their characteristics.
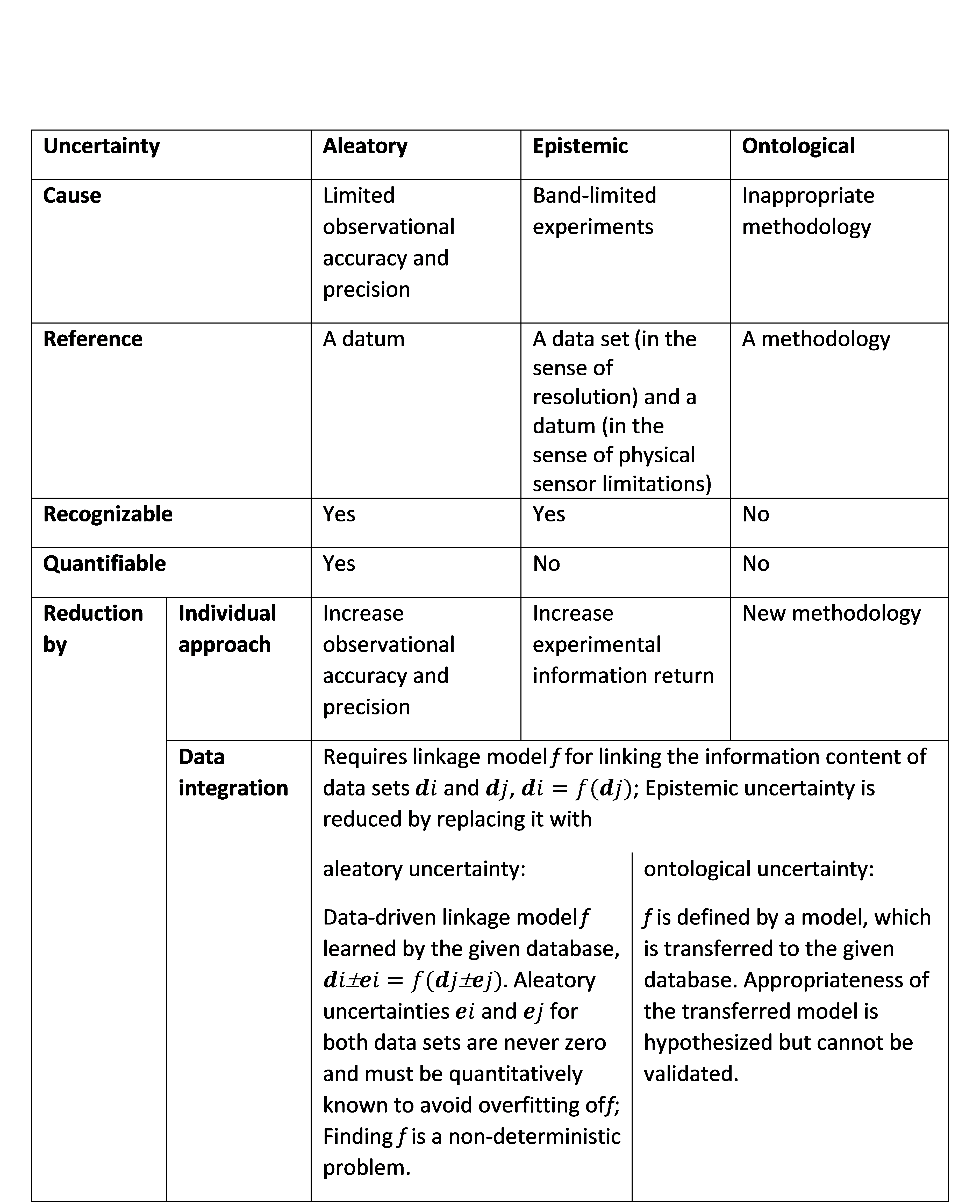
Fig. 75 Types of uncertainty ([Paasche et al., 2022], modified).#
Semantic Uncertainty and its Relation to Subjectivity#
Among others, [Beven, 2016] defined semantic uncertainty and suggested it as uncertainty type in addition to those listed in Fig. 75. According to [Beven, 2016], semantic uncertainty refers to “what statements or quantities in the relevant domain actually mean. … This can partly result from commensurability issues that quantities with the same name have different meanings in different contexts or scales.” This definition refers highly to information retrieval in information processing chains and the uncertainties human agents may have to deal with when retrieving information from data with aleatory uncertainty (e.g., the inaccuracy and impreciseness of data), epistemic uncertainty (e.g., incomplete data lacking aleatory uncertainty or discrete data sets) and/or ontological uncertainty (e.g., the suitability of a chosen method at any stage from information coding to information retrieval). We consider semantic uncertainty as a cross-cutting uncertainty type linked to information retrieval aspects in relation to any of the uncertainty types in Fig. 75. Since epistemic and ontological uncertainty are generally unquantifiable, information retrieval is challenging from data or data sets with such kinds of uncertainty. If no additional data are available to reduce information gaps in data, it requires the filling of information gaps resultant from epistemic uncertainty using a methodology whose appropriateness can only be assumed, but not quantified. The uncertainty of the chosen methodology is related to the quality of being based on or influenced by personal feelings, tastes, or opinions defining subjectivity and a human agents personality trait. This can be influenced by a (scientific) group’s feelings, tastes or opinions without necessarily losing an individual note. Ontological uncertainty cannot be expected to vanish, even when the agent is experienced and skilled, since human agents only hold a limited view on the world [Hanea et al., 2022]. Subjectivity and semantic uncertainty may not only occur at the information retrieval step. If human agents use their senses for sampling a phenomenon, e.g., in the environmental reality around them, the ability to objectively quantify the aleatory uncertainty of the produced data is compromised pretty early in the information processing chain.
References#
- Bev16(1,2)
Keith Beven. Facets of uncertainty: epistemic uncertainty, non-stationarity, likelihood, hypothesis testing, and communication. Hydrological Sciences Journal, 61(9):1652–1665, 2016.
- DKD09(1,2)
Armen Der Kiureghian and Ove Ditlevsen. Aleatory or epistemic? does it matter? Structural safety, 31(2):105–112, 2009.
- HHN22(1,2)
Anca M Hanea, Victoria Hemming, and Gabriela F Nane. Uncertainty quantification with experts: present status and research needs. Risk Analysis, 42(2):254–263, 2022.
- JCG08(1,2)
JCGM. Evaluation of measurement data – guide to the expression of uncertainty in measurement. Joint Committee for Guides in Metrology, 2008.
- LM05
David A Lane and Robert R Maxfield. Ontological uncertainty and innovation. Journal of evolutionary economics, 15:3–50, 2005.
- PGLuttgau+22(1,2)
Hendrik Paasche, Matthias Gross, Jakob Lüttgau, David S Greenberg, and Tobias Weigel. To the brave scientists: aren't we strong enough to stand (and profit from) uncertainty in earth system measurement and modelling? Geoscience Data Journal, 9(2):393–399, 2022.
- PPD20(1,2,3)
Hendrik Paasche, Katja Paasche, and Peter Dietrich. Uncertainty as a driving force for geoscientific development. Nature and Culture, 15(1):1–18, 2020.