Lévy Processes (DRAFT)#
Part of a series: Stochastic fundamentals.
Follow reading here
An important class of stochastic processes is given by Lévy processes. They provide a flexible framework for processes with stationary and independent increments that allows for continuous processes as well as processes with jump discontinuities. For example, it encompasses well-known processes like Brownian motion and Poisson processes.
Definition#
For the rest of this article, it is assumed that the underlying probability space is denoted by \((\Omega,\mathcal{F},\mathbb{P})\) and \(\mathbb{F}=(\mathcal{F}_t)_{t\ge0}\) is a filtration that is assumed to be right-continuous and complete.
An adapted stochastic process \(X=(X_t)_{t\ge0}\) on \(\mathbb{R}\) is a (one-dimensional) Lévy process if the following conditions are satisfied:
\(X_0=0~\mathbb{P}\)-almost surely.
For any \(n\in\mathbb{N}\) and \(0\le t_0<t_1<\dots<t_n<\infty\), the random variables \(X_{t_0},X_{t_1}-X_{t_0},\dots,X_{t_n}-X_{t_{n-1}}\) are independent.
\(X_{s+t}-X_s\stackrel{d}{=}X_t\) for \(0\le s,t<\infty\).
\(X\) is continuous in probability, i.e. for every \(t\ge0\) and \(\varepsilon>0\), it holds that
Condition 2 is called the “independent increments property”. It means that the behaviour of a Lévy process is independent along different time intervals. The “stationary increments property” 3 implies that, probabilistically, a process behaves equally in every time interval with the same length [Applebaum, 2009].
Properties#
In this section, some characteristic properties of Lévy processes are collected [Protter, 2004].
The characteristic function of a Lévy process \(X\) is determined by the so-called Lévy-Khintchine formula. For \(t\ge0\), it states that
\[ \begin{align*} \phi_{X_t}(u)=\mathbb{E}\left[\mathrm{e}^{-\mathrm{i}uX_t}\right]=\mathrm{e}^{-t\psi(u)},\quad u\in\mathbb{R}, \end{align*} \]where the function \(\psi\colon\mathbb{R}\to\mathbb{C}\) is of the form
\[ \begin{align*} \psi(u)=\frac{\sigma^2}{2}u^2-\mathrm{i}\gamma u+\int_{\mathbb{R}\setminus\{0\}}\left(1-\mathrm{e}^{-\mathrm{i}ux}+\mathrm{i}ux\mathbb{1}_{\{|x|<1\}}(x)\right)\nu(\mathrm{d}x), \end{align*} \]where \(\sigma\ge0,~\gamma\in\mathbb{R}\) and \(\nu\) is a measure on \(\mathbb{R}\setminus\{0\}\) satisfying
\[ \begin{align*} \int_{\mathbb{R}\setminus\{0\}}(1\wedge x^2)\nu(\mathrm{d}x)<\infty. \end{align*} \]A Lévy process is uniquely characterised by its corresponding parameters \(\sigma,~\gamma\) and \(\nu\). In particular, \(\gamma\) describes the drift of such a process, \(\sigma\) represents the Brownian motion component and \(\nu\) is the Lévy measure determining jump intensities. The triplet \((\gamma,\sigma^2,\nu)\) is referred to as the generating triplet.
Every Lévy process \(X\) can assumed to be càdlàg (i.e. the paths \(t\mapsto X_t\) are right-continuous \(\mathbb{P}\)-a.s. and the left limits exist \(\mathbb{P}\)-a.s.).1
If there exists a constant \(C>0\) such that \(\sup_{0\le t<\infty}|\Delta X_t|<C\), where \(\Delta X_t=X_t-X_{t-}\) with \(X_{t-}=\lim_{s\uparrow t}X_s\), then \(\mathbb{E}[|X_t|^n]<\infty\) for every \(t\ge0\) and \(n\in\mathbb{N}\).
Lévy processes are examples of Markov processes and semimartingales.
Examples#
By concretely determining distributions for the stationary increments property 3, different kinds of Lévy processes arise [Bass, 2011, Applebaum, 2009]). Corresponding paths are depicted in Fig. 15.
Brownian Motion#
One of the most prominent stochastic processes is Brownian motion. A process \(B=(B_t)_{t\ge0}\) is a Brownian motion if it is a Lévy process and if \(B_t\sim\mathcal{N}(0,t),~t\ge0\). It can also be seen as an example of a Markov process.
The generating triplet of a Brownian motion is given by \((\gamma,\sigma^2,\nu)=(0,1,0)\). In the context of Lévy processes, a Brownian motion \((B_t)_{t\ge0}\) exhibits some interesting path properties:
The paths \(t\mapsto B_t\) are continuous \(\mathbb{P}\)-a.s.
The paths \(t\mapsto B_t\) are \(\mathbb{P}\)-a.s. nowhere differentiable.
The continuity of the paths is a particular strong property since the paths of a Lévy process are in general only càdlàg.
Poisson Process#
A Poisson process is a typical pure jump process whose name stems from the fact that the increments are Poisson distributed. More formally, a process \(N=(N_t)_{t\ge0}\) is said to be a Poisson process with parameter \(\lambda>0\) if it is a Lévy process and if \(N_t\sim Pois(\lambda t),~t>0\). Here, Poisson processes are discussed as examples of Markov processes.
The generating triplet is \((\gamma,\sigma^2,\nu)=(0,0,\lambda\delta_1)\), where \(\delta_x\) denotes the Dirac measure on \(\mathbb{R}\) for given \(x\in\mathbb{R}\). Furthermore, it can be shown that the paths of a Poisson process are increasing and constant except for jumps of size \(1~\mathbb{P}\)-a.s.
Compound Poisson Process#
Compound Poisson processes are also pure jump processes, but they generalize Poisson processes by enabling processes to have arbitrary jump sizes.
For a mathematical definition, let \(N\) be a Poisson process with parameter \(\lambda>0\) and let \((Z_k)_{k\in\mathbb{N}}\) be a sequence of i.i.d. random variables taking values in \(\mathbb{R}\) with common law \(\mu_Z\), where \(\mu_Z\) is a distribution on \(\mathbb{R}\) with \(\mu_Z(\{0\})=0\). It is supposed that \(N\) is independent of all \(Z_k\)’s. A compound Poisson process \(Y=(Y_t)_{t\ge0}\) is then defined as
The generating triplet of such a compound Poisson process is
As already mentioned, the definition of a compound Poisson processes allows for random jump sizes. They can take all values supported by the distribution \(\mu_Z\). If \(\mu_Z=\lambda\delta_1\), for \(\lambda>0\), a compound Poisson process reduces to a Poisson process with parameter \(\lambda\).
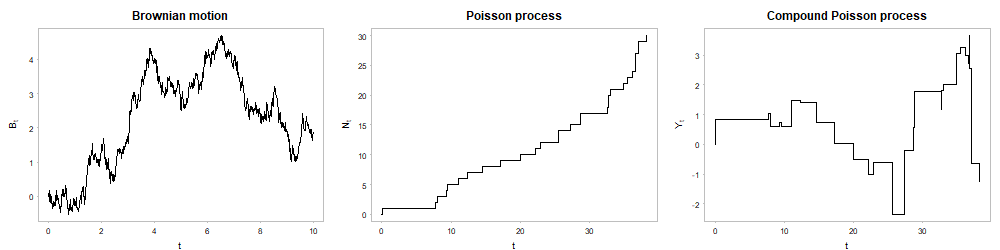
Fig. 15 Paths of a Brownian motion, a Poisson process and a compound Poisson process#
Literature#
- App09(1,2)
David Applebaum. Lévy Processes and Stochastic Calculus. Volume 116 of Cambridge Studies in Advanced Mathematics. Cambridge University Press, Cambridge, 2. ed. edition, 2009.
- Bas11
Richard F. Bass. Stochastic processes. Volume 33 of Cambridge series in statistical and probabilistic mathematics. Cambridge University Press, Cambridge, 1 edition, 2011.
- Pro04
Philip E. Protter. Stochastic Integration and Differential Equations. Volume 21 of Stochastic Modelling and Applied Probability. Springer-Verlag, Berlin, 2. ed. edition, 2004.
Contributors#
Philipp Böttcher
- 1
More precisely, for any Lévy process, there exists a unique modification which is càdlàg \(\mathbb{P}\)-a.s. and which is also a Lévy process.