Modelling The Degradation Of Biodegradable Implants#
Part of a series: Uncertainty quantification in Implants Materials.
Follow reading here
Various mathematical models have been developed for the corrosion of metallic systems. This enables the computational models to be used as a research tool to improve understanding of this process. In the case of biodegradable implants, these implants must decay at a rate that matches the healing rate of biological tissues. This degradation must occur with no toxic residues left behind [Esmaily et al., 2017]. Therefore, controlling the degradation is one of the keys in developing biodegradable implants. However, modelling this is a very challenging process due to the complex nature of biodegradation process of Mg-based implants under physiological conditions. Biodegradable Mg-based implants have been studied using a variety of computational models, including physical, phenomenological, analytical models and machine-learning algorithms [Abdalla et al., 2020, Albaraghtheh et al., 2022, Ansari et al., 2019, Boland et al., 2016, Zhang and Hao, 2021]. In [Albaraghtheh et al., 2022] we provide a detailed discussion of these modelling approaches and highlight their added values and drawbacks.
Challenges in Modelling the Degradation of Mg-based Implants#
The main challenges in modelling the degradation of Mg-based implants can be summarized as: the need to have a thorough understanding of degradation and the factors affecting it. However, the precise mechanisms of the degradation are still unclear [Gonzalez et al., 2018, Mei et al., 2020]. Several factors affect the degradation process as summarized by the schematic diagrams in Fig. 78. These factors are pH, temperature, hydrodynamic conditions, the degradation media composition and alloying elements. Details of how these factors are contributing to the degradation process are summarized in [Albaraghtheh et al., 2022]. Under physiological conditions, these physical, chemical, biological factors and their interactions increase the complexity of the mathematical models of Mg-based implants and introduce the dimensionality challenge to the modelling process. Moreover, in the in vivo case, where the implant is tested within an animal model, the complexity of the process increases; for instance, the presence and different reactions of cells are observed in this setting. [Esmaily et al., 2017].
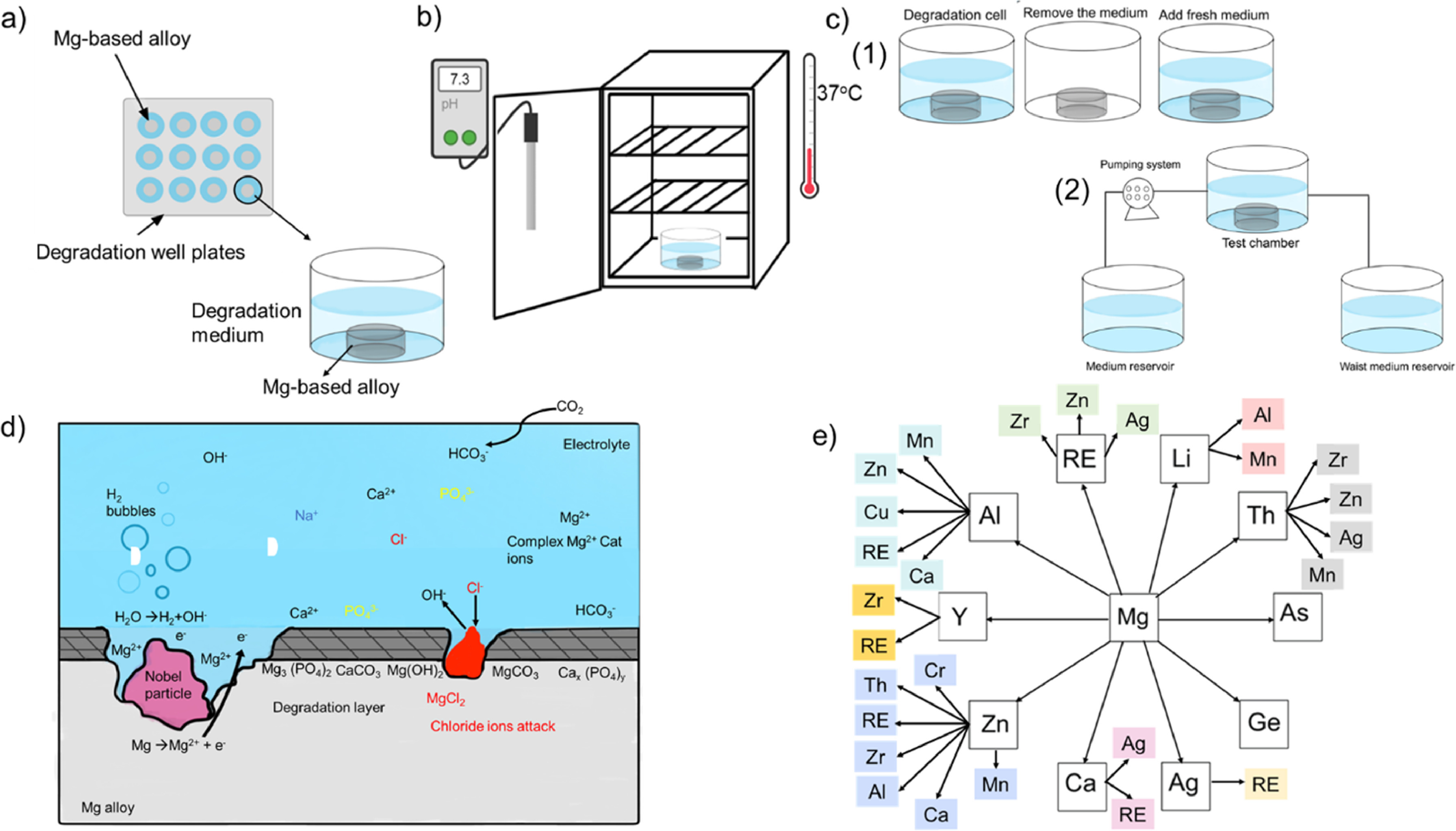
Fig. 78 Schematic diagram of the key factors affecting the degradation of Mg-based alloys in physiological media. (a) Schematic diagram in vitro experiment set up. (b) Schematic diagram of controlling the pH and Temperature during the in vitro test within the incubator. (c) Schematic diagram of the hydrodynamic conditions, (1) static and (2) dynamic set in vitro test. (d) Schematic diagram of media composition of the degradation of Mg-based alloys. (e) Most reported alloying elements for developing Mg alloys. (adapted under CC-BY-4.0 license [Albaraghtheh et al., 2022]).#
Moreover, the degradation models must take into account not only the Mg surface and the physiological environment, but also the formation of degradation products [Albaraghtheh et al., 2022, Barzegari et al., 2021, Gonzalez et al., 2018, Xin et al., 2008, Yang et al., 2020]. The degradation products form a porous degradation layer between the implant surface and the degradation media, as can be seen in Fig. 79 b . Also, the model should consider the medium flow, which means whether the experiments are taking place under static or dynamic conditions. Moreover, the species distribution and ionic content of the degradation media should be taken into account. For example, modelling pure Mg under physiological conditions involves several chemical reactions other than the degradation reactions that occur on the implant surface. This can be seen in Fig. 79 a.
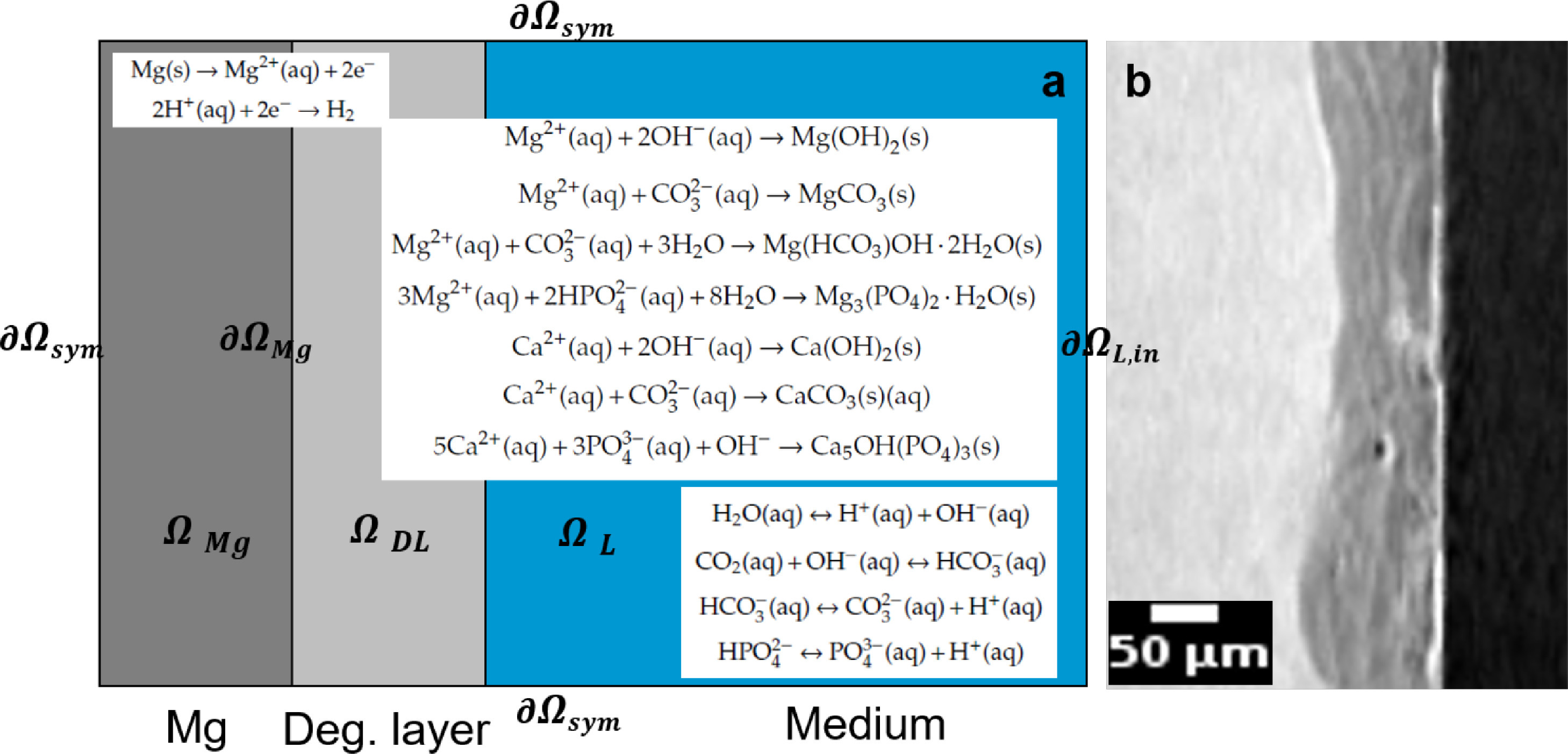
Fig. 79 a Schematic of the degradation process of pure Mg in simulated body fluid. The metallic Mg sample is assumed to be covered by a porous degradation layer from the start, with an initial thickness of 10 nm. The ions diffuse through the liquid medium and the liquid phase of the degradation layer towards the metal interface, where the Mg dissolution is taking place. Precipitates form within the degradation layer and the dissociation of different ionic species occurs in the liquid phase. Not to scale. b Slice through µCT data of pure Mg disc degraded in SBF after 3 weeks for comparison. (adapted under CC-BY-4.0 license [Zeller-Plumhoff et al., 2022]).#
In order to overcome the dimensionality problem, degradation models often make simplified assumptions or use reductionist approaches, such as considering only one or two dimensional aspects of the degradation process [Milone and Landolfo, 2022]. However, there is a possibility that these simplified models are not accurate at capturing the multidimensional, complex nature of degradation. In addition, they are ineffective at predicting degradation behavior in reality. Modeling the degradation of Mg-based implants is a complex process that needs to overcome the challenges of accurately capturing the multidimensional and complex nature of degradation within a reasonable computational timeframe. It is inevitable that not addressing these challenges correctly will produce uncertain and unreliable predictions of degradation behavior. This can limit the usefulness of degradation models in actual applications. To enhance the reliability of degradation models and to to overcome these challenge, the uncertainty quantification (UQ) approach provides methods and algorithms to quantify uncertainties of different types, natures and sources. Nevertheless, it is very time-consuming to quantify these uncertainties, particularly using sampling-based methods, due to the repeated evaluations of highly accurate approximations of the complex models involved. A robust solution to this challenge is implementing a surrogate modeling approach under uncertainty, in which complex degradation models are approximated by easy-to-evaluate mathematical functions.
References#
- AJEI20
Moataz Abdalla, Alexander Joplin, Mohammad Elahinia, and Hamdy Ibrahim. Corrosion modeling of magnesium and its alloys for biomedical applications. Corrosion and Materials Degradation, 1(2):11, 2020.
- AWRomerZP22(1,2,3,4,5)
Tamadur Albaraghtheh, Regine Willumeit-Römer, and Berit Zeller-Plumhoff. In silico studies of magnesium-based implants: a review of the current stage and challenges. Journal of Magnesium and Alloys, 2022.
- ALS19
Talha Qasim Ansari, Jing-Li Luo, and San-Qiang Shi. Modeling the effect of insoluble corrosion products on pitting corrosion kinetics of metals. NPJ Materials Degradation, 3(1):28, 2019.
- BMLG21
Mojtaba Barzegari, Di Mei, Sviatlana V Lamaka, and Liesbet Geris. Computational modeling of degradation process of biodegradable magnesium biomaterials. Corrosion Science, 190:109674, 2021. URL: https://www.sciencedirect.com/science/article/pii/S0010938X21004406, doi:https://doi.org/10.1016/j.corsci.2021.109674.
- BSK+16
Enda L Boland, Connor J Shine, Nicola Kelly, Caoimhe A Sweeney, and Peter E McHugh. A review of material degradation modelling for the analysis and design of bioabsorbable stents. Annals of biomedical engineering, 44:341–356, 2016.
- ESF+17(1,2)
M Esmaily, JE Svensson, S Fajardo, N Birbilis, GS Frankel, S Virtanen, R Arrabal, S Thomas, and LG Johansson. Fundamentals and advances in magnesium alloy corrosion. Progress in Materials Science, 89:92–193, 2017.
- GHN+18(1,2)
Jorge Gonzalez, Rui Qing Hou, Eshwara PS Nidadavolu, Regine Willumeit-Römer, and Frank Feyerabend. Magnesium degradation under physiological conditions–best practice. Bioactive materials, 3(2):174–185, 2018.
- MLLZ20
Di Mei, Sviatlana V Lamaka, Xiaopeng Lu, and Mikhail L Zheludkevich. Selecting medium for corrosion testing of bioabsorbable magnesium and other metals–a critical review. Corrosion Science, 171:108722, 2020.
- ML22
Aldo Milone and Raffaele Landolfo. A simplified approach for the corrosion fatigue assessment of steel structures in aggressive environments. Materials, 15(6):2210, 2022.
- XHT+08
Yunchang Xin, Kaifu Huo, Hu Tao, Guoyi Tang, and Paul K Chu. Influence of aggressive ions on the degradation behavior of biomedical magnesium alloy in physiological environment. Acta biomaterialia, 2008.
- YHD+20
Youwen Yang, Chongxian He, E Dianyu, Wenjing Yang, Fangwei Qi, Deqiao Xie, Lida Shen, Shuping Peng, and Cijun Shuai. Mg bone implant: features, developments and perspectives. Materials & Design, 185:108259, 2020.
- ZPAHocheWRomer22
Berit Zeller-Plumhoff, Tamadur AlBaraghtheh, Daniel Höche, and Regine Willumeit-Römer. Computational modelling of magnesium degradation in simulated body fluid under physiological conditions. Journal of magnesium and alloys, 10(4):965–978, 2022.
- ZH21
Xuanbin Zhang and Zhixiu Hao. Computational models of magnesium medical implants degradation: a review. In Journal of Physics: Conference Series, volume 1838, 012012. IOP Publishing, 2021.
Contributors#
Julian Wäsche, Berit Zeller-Plumhoff