Subjectivity in the Data Production - Geological Map Production as an Example#
This article is part of a series: Subjectivity in Earth Scinece Data.
Follow reading here
If human sensory engagement is involved in data production, the ability to produce aleatory uncertainty for the produced data is compromised. Expert elicitation or crowd annotation is often practically impossible or economically prohibitive and self-assessment of a human agent’s uncertainty does not overcome the problem of producing incomplete data lacking aleatory uncertainty. An example for this is geological mapping, in which skilled geologists inspect the Earth’s surface at sparse locations to produce geological map products displaying the distribution and characteristics of rock units, geological features, and structures in a particular geographic area. Geological maps are data representations using georeferenced colors and shapes for information coding. Realistic uncertainty quantification for geological maps or methodologically related data products, such as soil maps, is a matter of ongoing research. A solution to the problem might be rooted in information fusion and data integration.
Uncertainty Quantification in Geoscientific Imaging and Image Fusion#
Geoscientists explore the states of compartments of the Earth system and of the interaction of these systems with each other [Manduca and Kastens, 2012]. Studying state variables [Williams et al., 2012] and processes of the Earth relies on a broad portfolio of sensing technology used to produce images of the Earth and processes therein. Typical geoscientific products are maps imaging state variables of the Earth on a 2D plane.
A common geoscientific task is the generation of labeled images, for example the attribution of soil classes or geological classes in a geoscientific map. Still strongly relying on the human sense of sight, visual inspection by human experts is a frequently used and valuable source to produce labeled images (e.g., [Pestrong, 2000]), but inevitably generates subjectivity. The results of such endeavors, seen as visual measurements, come with truth and fallacies as well as variable and unknown trustworthiness. The identification of subjectivity in an image generation process and its resultant data, e.g., visualized as a geological map, cannot be done by considering only this image nor based on the information perception of the geoscientist, which is inherently subjective, too. Typically, subjectivity in the generation of map images becomes only visible at map sheet edges, i.e., if inconsistencies between two map sheets resulting from different mapping surveys become visible (Fig. 76). Beside label inconsistencies (e.g., as visible in Fig. 76), also other structural mismatches, such as border faults, may occur.
Despite the unknown fraction of subjectivity in geoscientific mapping products relying at least partly on the human sense of sight, the resultant maps are important layers of information, which have proven value over centuries, such as geological maps. While skilled human perceivers of such maps qualitatively account for the subjectivity-related uncertainty of such maps, numerical algorithms, e.g., laymen and machine learning algorithms, lack geoscientific expertise to correctly retrieve information from maps bearing subjectivity.
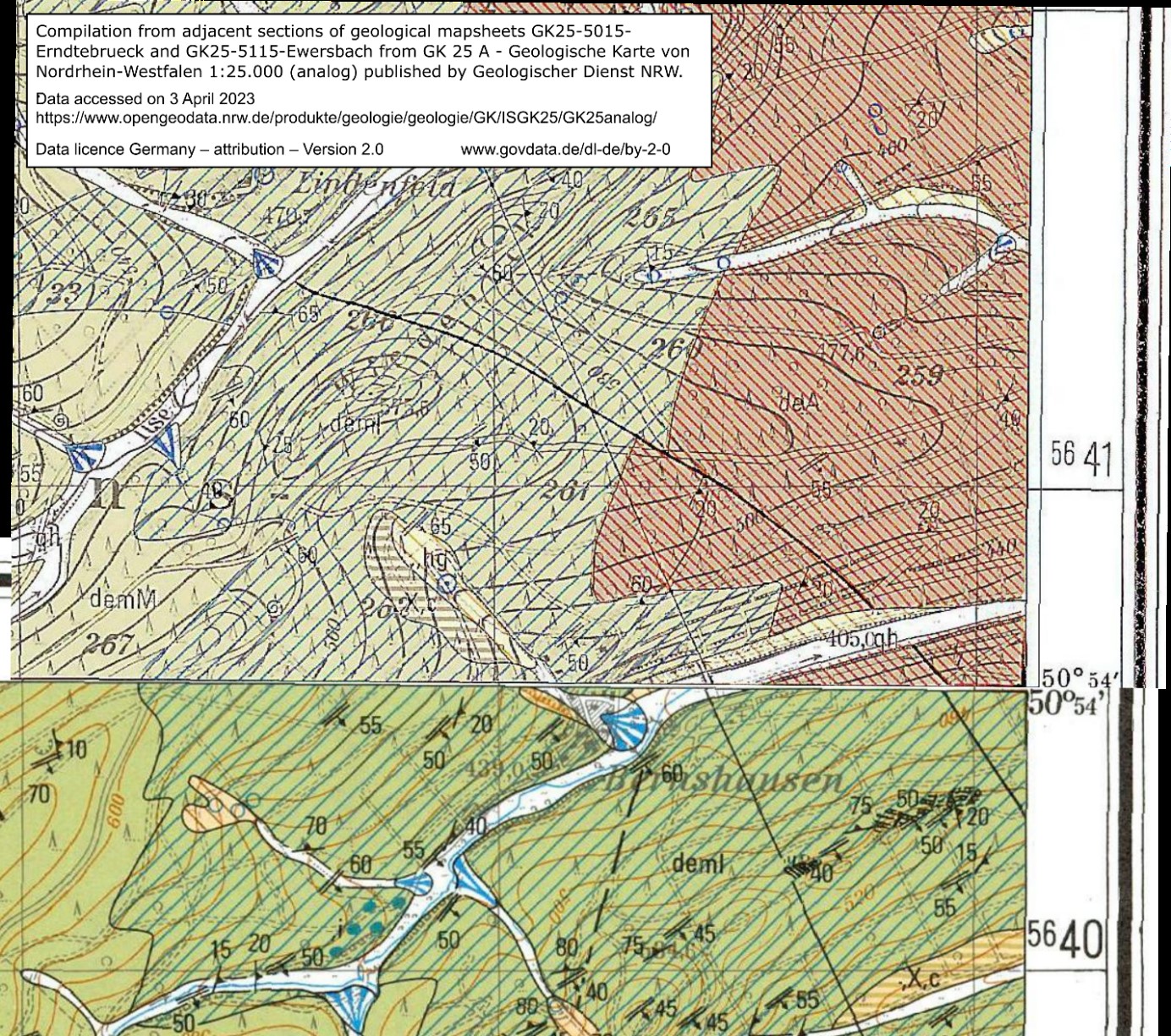
Fig. 76 Compilation from two geological map sheets with their map sheet edge at 50°54´ N. Note the different labels deml and deA at the map sheet edges indicating different rock units.#
Subjective vs. Technical Maps and their Uncertainty#
Usually, multiple co-located maps are available over a survey area. Some of these maps may result from data acquisition routines relying on technical sensors following algorithmic data recording procedures resulting in data, whose aleatory uncertainty is quantitatively known. Examples are geophysical maps or satellite imagery. This is a significant difference to partly subjective maps, for which aleatory uncertainty cannot be quantified by uncertainty propagation from the signal perceived by the receptors in the eye through the human information perception and data production.
However, if subjectively and technically sampled maps are co-located, they provide information about the same reality [Asadi et al., 2016]. Albeit the distinct relations between the maps may be unknown and spatially variable, variability of the mapped state variables indicates inhomogeneous ground conditions. Different sensitivities may exist, so that changing ground conditions may not result in a variability of the mapped state variable large enough to be sensed. While this does not allow to identify a priori a pair of maps that is most similar in its information content and therefore particularly suitable for a mutual information confirmation, it ensures that a comparison of a subjective map with many co-located technically sampled maps may converge towards a reliable information confirmation.
Treating subjectivity in maps as epistemic uncertainty, a kind of uncertainty a perceiver is aware of but which is not quantitatively known, it can be converted into aleatory, i.e., quantifiable uncertainty, by information fusion approaches [Paasche et al., 2020]. Prerequisite is, that the data sets, i.e., maps, to be fused with the partly subjective image, come with quantified, i.e., aleatory, uncertainty.
Utilization of the subjective image as a benchmark in the information fusion approach is prohibitive. Instead, unsupervised pattern matching fully crediting the aleatory uncertainty of technically sampled maps may allow to compute a quantitative estimate of the trustworthiness of the information coded in label images emanating from a subjective imaging procedure, i.e., as is the case for geological map generation. Any statistical pattern similarity measures capable to propagate data uncertainty of the technical maps through their analyses may be suitable to do the task.
References#
- ADP16
Abduljabbar Asadi, Peter Dietrich, and Hendrik Paasche. 2d probabilistic prediction of sparsely measured earth properties constrained by geophysical imaging fully accounting for tomographic reconstruction ambiguity. Environmental Earth Sciences, 75:1–15, 2016.
- MK12
Cathryn A Manduca and Kim A Kastens. Geoscience and geoscientists: uniquely equipped to study earth. Geological Society of America Special Papers, 486:1–12, 2012.
- PPD20
Hendrik Paasche, Katja Paasche, and Peter Dietrich. Uncertainty as a driving force for geoscientific development. Nature and Culture, 15(1):1–18, 2020.
- Pes00
Ray Pestrong. Geology–the sensitive science. Journal of Geoscience Education, 48(3):333–336, 2000.
- WBA+12
Kristen J Williams, Lee Belbin, Michael P Austin, Janet L Stein, and Simon Ferrier. Which environmental variables should i use in my biodiversity model? International Journal of Geographical Information Science, 26(11):2009–2047, 2012.
Authors#
Jalil Asadi